Professor Wu Junfeng's team from Hong Kong Chinese University (Shenzhen) - UWB ranging to realize mobile robot position and attitude estimation At 0.5m/s, the average positioning error of about 3cm and the average angle error of 4° are realized
The pose (position and attitude) estimation function of mobile robots is the basic prerequisite for achieving tasks such as obstacle avoidance and path planning. In recent years, researchers have been working to achieve high-performance and real-time state estimation using airborne sensors such as inertial measurement units (IMUs), cameras, and lidar. However, the position pose estimation module based on the onboard sensor is prone to long-term trajectory drift problems and is less robust in specific environments.
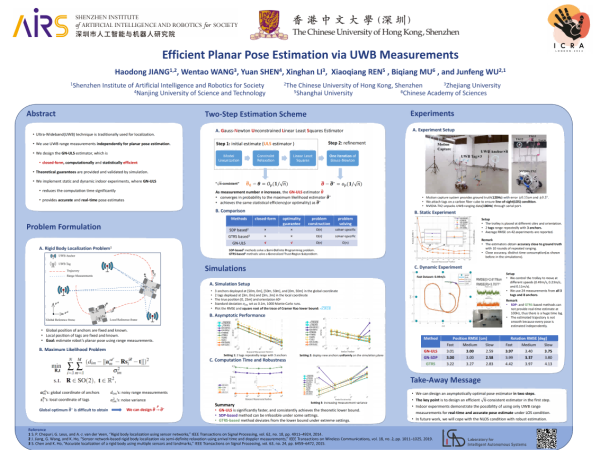
ICRA Poster
Recently, Professor Wu Junfeng's team from the School of Data Science of Hong Kong Chinese University (Shenzhen) published an article entitled Efficient Planar Pose Estimation via UWB Measurements at the top conference in robotics ICRA (IEEE International Conference on Robotics and Automation, one of the most authoritative conferences in the field of robotics). The distance measurement function of UWB ultra-wideband radio technology (LinkTrack UWB high-precision ranging and positioning) is used to realize real-time high-precision pose estimation of planar mobile robots, which effectively avoids the above problems. Experiment at 0.5m/s average speed: the average error of position estimation of the GN-ULS estimator is 3.01cm, and the average error of angle estimation is 3.97°. (Download link at the end of the article) ...
UWB technology is widely used in the positioning of mobile robots, but there are few jobs using UWB technology to estimate the pose of mobile robots. The team modeled this problem as a Rigid Body Localization (RBL) problem in the field of signal processing. Through literature research, the team found that the previous literature lacked statistical asymptotic validity analysis of estimators, and the computational complexity of the proposed algorithm was high.
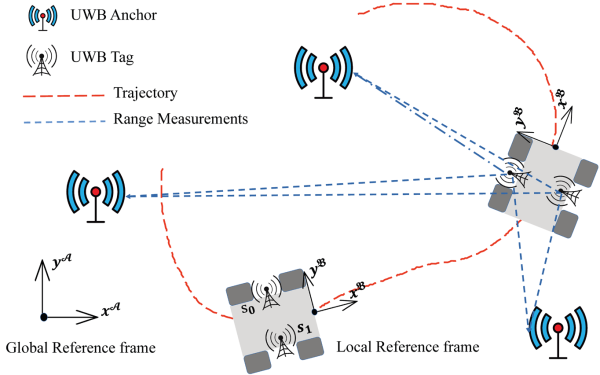
Schematic diagram of robot plane pose estimation based on UWB distance measurement
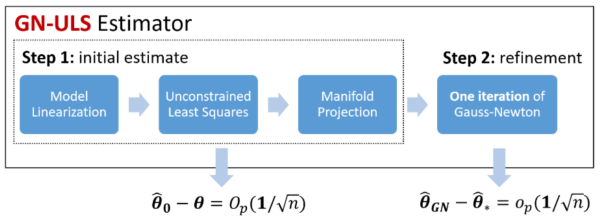
Two-step estimation framework
This work proposes the pose estimator GN-ULS based on a two-step method:
-
(1) We first relax the maximum likelihood problem into a linear least squares problem by transforming the observation equation and parametric rotation matrix, and prove that its least squares solution is a consistent estimate of the true pose -
(2) On this basis, we project the pose estimation obtained in the first step to a special orthogonal group, and improve the estimate by Gauss-Newton algorithm. We show that the results of the one-step Gauss-Newton algorithm converge to the maximum likelihood estimate.
In summary, the estimator proposed in this work has the following advantages: 1) with the increase of the number of distance measurements, the pose estimation converges to the true pose at the theoretical fastest rate (that is, the mean squared error reaches the lower bound of Kramero); 2) Compared with previous studies, the computational complexity has been significantly improved, and the real-time solution of dynamic pose has been realized; 3) The covariance matrix of pose estimation is given. In addition, this study also discusses and studies the observability of plane pose estimation problems of mobile robots based on distance measurement, and uses graph rigidity theory to give sufficient conditions for observability.
In simulation experiments, we test the performance of different estimators under different sensor numbers, repeated observations, and noise variance. The result is shown in the following figure. The GN-ULS estimator proposed in this study can reach the lower bound of the theoretical optimal under different simulation settings, while the previous methods will deviate from the lower bound of the theoretical optimal in specific scenarios.
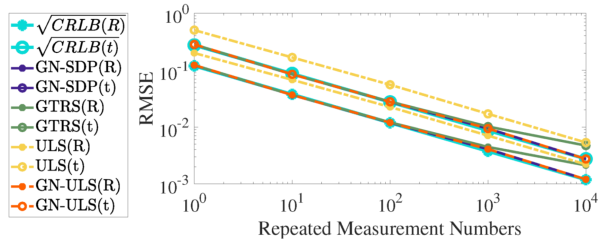
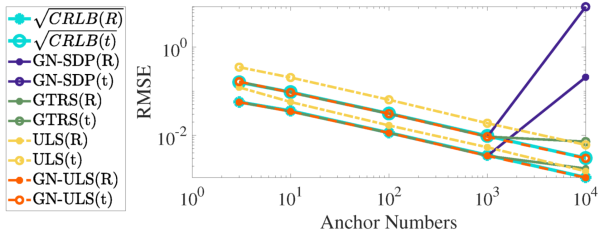
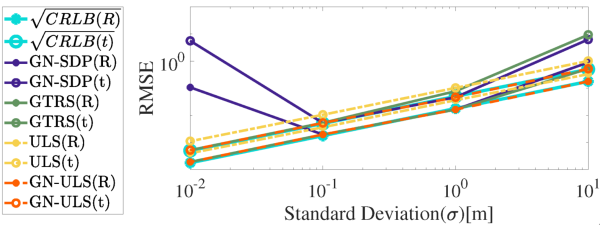
Simulation experiment results
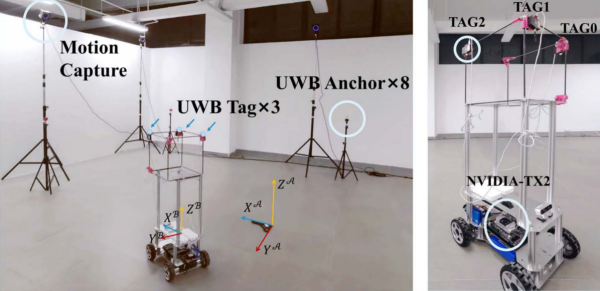
Experimental environment and trolley experimental platform
In the real scenario, we built a trolley experimental platform based on hardware equipment such as NVIDIA-TX2, used the motion capture system to provide the real value of the trolley pose in real time, and collected UWB distance measurement data in static and dynamic scenarios, and the experimental environment and trolley platform are shown in the figure below.
Due to the obstruction of obstacles and wall reflections in the indoor environment, the UWB distance measurement data in the real experiment is affected by the outlier, and we exclude the measurement outlier by setting a threshold for the change of the distance measurement at adjacent time in the experiment. We collected 42 sets of static measurement data in different positions and postures, and the experimental results are shown in the figure below. In this experiment, the variance of the noise is millimeters, and the accuracy of the proposed GN-ULS estimator is similar to that of other methods, and its advantages are reflected in the computational complexity: GN-ULS can process tens of thousands of distance measurements in 1 millisecond; The time consumption of the previous method is about 0.1s.
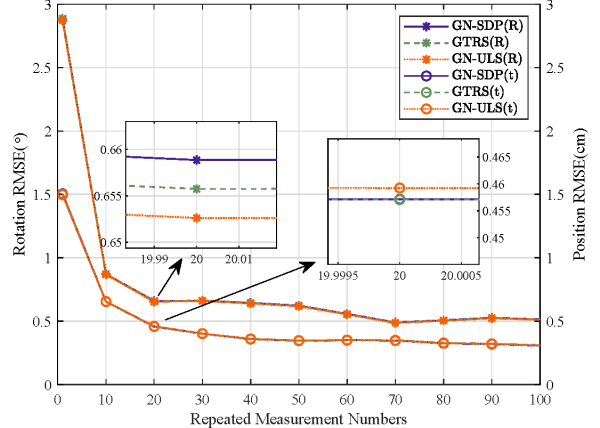
Static pose estimation experimental results
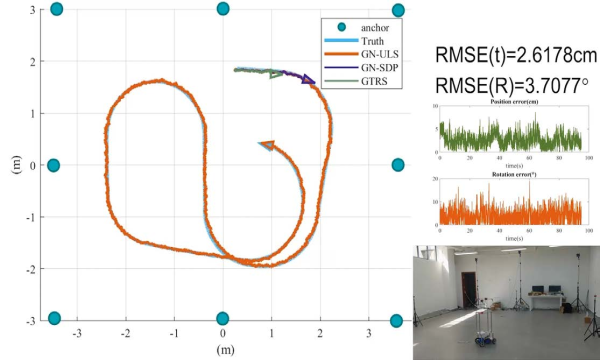
Dynamic pose estimation experimental results
We controlled the trolley to move in the indoor environment at an average speed of 0.5m/s, 0.2m/s, 0.1m/s, and collected UWB distance measurement data and pose true values. The experimental results at an average speed of 0.5m/s are shown in the figure below, the average error of position estimation of the GN-ULS estimator is 3.01cm, and the average error of angle estimation is 3.97°. The accuracy and smoothness of the estimated trajectory are expected to be further improved by combining odometry measurements with motion models.
In this work, the problem of robot plane pose estimation based on UWB distance measurement is studied. Based on the two-step method, we design a pose estimator with asymptotically efficient and low computational complexity, GN-ULS. Simulations and experiments show that GN-ULS is superior to previous work in terms of computational efficiency, robustness, and accuracy in noisy environments. In this work, we demonstrate the possibility of real-time, accurate estimation of robot pose using distance measurements alone.
-
Paper Link:https://arxiv.org/abs/2209.06779 -
Materials provided by Dr. Haodong Jiang